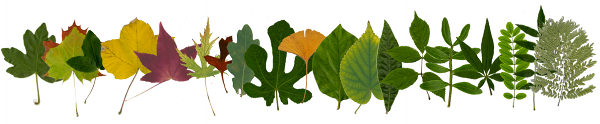
News |
A direct link to the overview of the task:
TThe ImageCLEF 2012 plant image identification task, CLEF 2012 working notes, Goëau H., Bonnet P., Joly A., Yahiaoui I., Barthelemy D., Boujemaa N., Molino, ImageCLEF 2012 working notes, Rome, Italy |
Dataset:
A public package containing the data of the 2012 plant retrieval task is now available (including the ground truth, an executable to compute scores, the working notes and presentations at ImageCLEF2012 Workshop, and additional informations). The entire data is under Creative Common license.
|
Context
If agricultural development is to be successful and biodiversity is to be conserved, then accurate knowledge of the identity, geographic distribution and uses of plants is essential. Unfortunately, such basic information is often only partially available for professional stakeholders, teachers, scientists and citizens, and often incomplete for ecosystems that possess the highest plant diversity. So that simply identifying plant species is usually a very difficult task, even for professionals (such as farmers or wood exploiters) or for the botanists themselves. Using image retrieval technologies is nowadays considered by botanists as a promising direction in reducing this taxonomic gap. Evaluating recent advances of the IR community on this challenging task might therefore have a strong impact. The organization of this task is funded by the French project Pl@ntNet (INRIA, CIRAD, Telabotanica) and supported by the European Coordination Action CHORUS+.

Task Overview
Following the success of ImageCLEF 2011 plant identification task, we are glad to organize this year a new challenge dedicated to botanical data. In the continuity of last year pilot, the task will be focused on tree species identification based on leaf images. The main novelties compared to last year are the following:
- more species: the number of species will be this year 126, which is an important step towards covering the entire flora of a given region.
- plant species retrieval vs. pure classification: last year's task was organized as a pure classification task over 70 species, whereas this year evaluation metric will be slightly modified in order to consider a ranked list of retrieved species rather than a single brute determination.
Until then, you can start experimentations with the ImageCLEF2011 Plant Task Final Package included in the next training data of the 2012 campaign.
ALL DATA of last year pilot task (training AND test) will be included in the training data of the 2012 campaign. This training data will be extended by the new contents collected through the citizen sciences initiative that was initiated last year in collaboration with Telabotanica (social network of amateur and expert botanists). The description of the crowdsourcing system used for creating these contents has been published in ACM Multimedia 2011 proceedings (online version). This process makes the task closer to the conditions of a real-world application: (i) leaves of the same species are coming from distinct trees living in distinct areas (ii) pictures and scans are taken by different users that might not used the same protocol to collect the leaves and/or acquire the images (iii) pictures and scans are taken at different periods in the year.
Additional information will still include contextual meta-data (author, date, locality name) and some EXIF data. Three types of image content will still be considered for training: leaf scans, leaf pictures with a white uniform background (referred as scan-like pictures) and leaf photographs in natural conditions (most of them taken on the tree).
Dataset
The task will be based on Pl@ntLeaves II dataset which focuses on 126 tree species from French Mediterranean area. It contains 11572 pictures subdivided into 3 different kinds of pictures: scans (57%), scan-like photos (24%) and free natural photos (19%). All data are published under a creative commons license. The following figure provides examples of the three categories
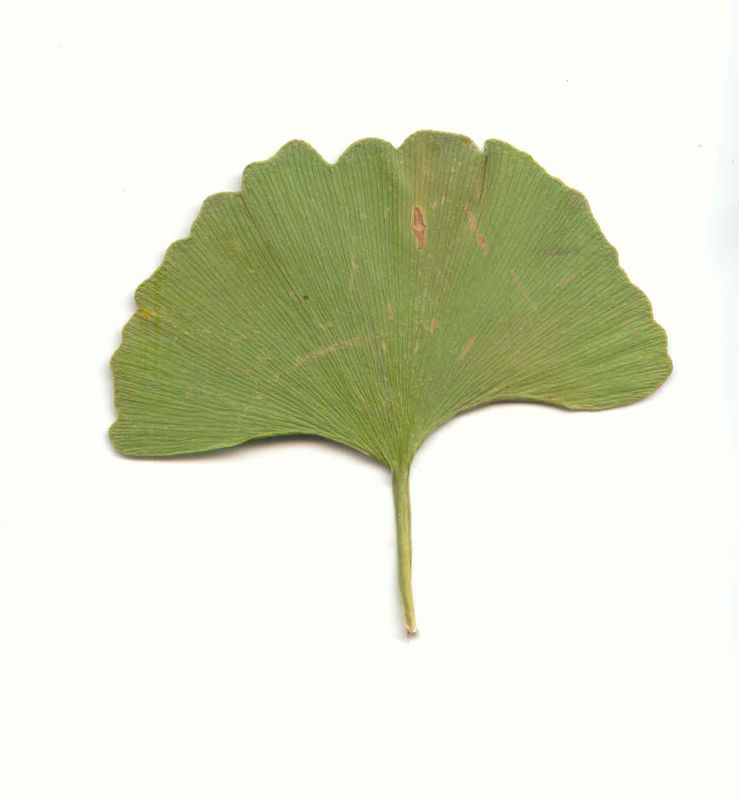
Scans of Boxelder Maple, London Plane, Holly, Paper Mulberry, Kaki Persimmon and Gingko

Scan-like pictures of Boxelder Maple, London Plane, Holly, Paper Mulberry, Kaki Persimmon and Gingko
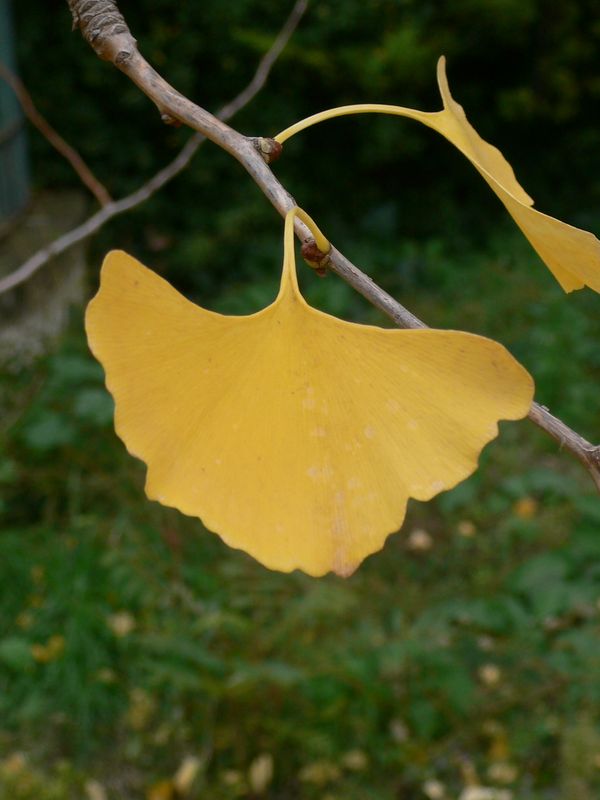
Photographs of Boxelder Maple, London Plane, Holly, Paper Mulberry, Kaki Persimmon and Gingko
Each image is associated with the following meta-data:
- date
- acquisition type: Scan, pseudoscan or photograph
- content type: single leaf, single dead leaf or foliage (several leaves on tree visible in the picture)
- full taxon name (species, genus, family…)
- English vernacular name (the common name),
- name of the author of the picture,
- name of the organization of the author
- locality name (a district or a country division or a regions).
- GPS coordinates of the observation
These meta-data are stored in independent xml files, one for each image. We provide here a set of 3 images (one of each type) and associated xml files :
1259.jpg 1259.xml
1817.jpg 1817.xml
1259.jpg 1259.xml
Partial meta-data information can be found in the image's EXIF, and might include:
- the camera or the scanner model,
- the image resolutions and the dimensions,
- for photos, the optical parameters, the white balance, the light measures…
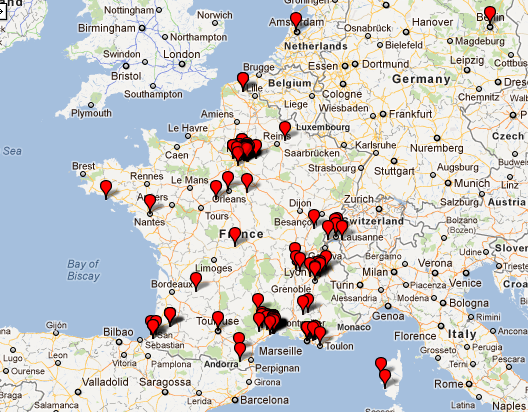
Localities in the ImageCLEF 2012 Plant Task dataset
Task description
The task will be evaluated as a plant species retrieval task.
training and test data
A part of Pl@ntLeaves II dataset will be provided as training data whereas the remaining part will be used later as test data. The training subset was built by including the training AND test subsets of last year Pl@ntLeaves dataset, and by randomly selecting 2/3 of the individual plants for each NEW species (several pictures might belong to the same individual plant but cannot be split across training and test data).
- The training data finally results in 8422 images (4870 scans, 1819 scan-like photos, 1733 natural photos) with full xml files associated to them (see previous section for few examples). A ground-truth file listing all images of each species will be provided complementary. Download link of training data will be sent to participants on March 26th.
- The test data results in 3150 images (1760 scans, 907 scan-like photos, 483 natural photos) with purged xml files (i.e without the taxon information that has to be predicted).
goal
The goal of the task is to retrieve the correct species among the top k species of a ranked list of retrieved species for each test image. Each participant is allowed to submit up to 3 runs built from different methods. Semi-supervised and interactive approaches, particularly for segmenting leaves from the background, are allowed but will be compared independently from fully automatic methods. Any human assistance in the processing of the test queries has therefore to be signaled in the submitted runs (see next section on how to do that).
run format
The run file must be named as "teamname_runX.run" where X is the identifier of the run (i.e. 1,2 or 3). The run file has to contain as much lines as the total number of predictions, with at least one prediction per test image and a maximum of 126 predictions per test image (126 being the total number of species). Each prediction item (i.e. each line of the file) has to respect the following format :
<test_image_name.jpg;ClassId;rank;score>
The ClassId is the pair <Genus_name_without_author_name Species_name_without_author_name> and forms a unique identifier of the species. These strings have to respect the format provided in the ground-truth file provided with training set (i.e. the same format as the fields <ClassId> in the xml metadata files, see examples in previous section). <rank> is the ranking of a given species for a given test image. <Score> is a confidence score of a prediction item (the lower the score the lower the confidence). Here is a fake run example respecting this format:
myteam_run2.txt
The order of the prediction items (i.e. the lines of the run file) has no influence on the evaluation metric, so that contrary to our example prediction items might be sorted in any way. On the other side, the <rank> field is the most important one since it will be used as the main key to sort species and compute the final metric.
For each submitted run, please give in the submission system a description of the run. A combobox will specify wether the run was performed fully automatically or with a human assistance in the processing of the queries. Then, a textarea should contain a short description of the used method, particularly for helping differentiating the different runs submitted by the same group, for instance:
matching-based method using SIFT features, RANSAC algorithm and K-NN classifier with K=10
Optionally, you can add one or several bibtex reference(s) to publication(s) describing the method more in details.
metric
The primary metric used to evaluate the submitted runs will be a score related to the rank of the correct species in the list of retrieved species. Each test image will be attributed with a score between 0 and 1 : of 1 if the 1st returned species is correct and will decrease quickly while the rank of the correct species increases. An average score will then be computed on all test images. A simple mean on all test images would however introduce some bias. Indeed, we remind that the Pl@ntLeaves dataset was built in a collaborative manner. So that few contributors might have provided much more pictures than many other contributors who provided few. Since we want to evaluate the ability of a system to provide correct answers to all users, we rather measure the mean of the average classification rate per author. Furthermore, some authors sometimes provided many pictures of the same individual plant (to enrich training data with less efforts). Since we want to evaluate the ability of a system to provide the correct answer based on a single plant observation, we also have to average the classification rate on each individual plant. Finally, our primary metric is defined as the following average classification score S:
U : number of users (who have at least one image in the test data)
Pu : number of individual plants observed by the u-th user
Nu,p : number of pictures taken from the p-th plant observed by the u-th user
Su,p,n : score between 1 and 0 equals to the inverse of the rank of the correct species (for the n-th picture taken from the p-th plant observed by the u-th user)
To isolate and evaluate the impact of the image acquisition type (scan, scan-like, natural pictures), an average classification score S will be computed separately for each type. Participants are allowed to train distinct classifiers, use different training subset or use distinct methods for each data type.
How to register for the task
ImageCLEF has its own registration interface. Here you can choose a user name and a password. This registration interface is for example used for the submission of runs. If you already have a login from the former ImageCLEF benchmarks you can migrate it to ImageCLEF 2011 here
___________________________________________________________________________________________________________________
Results
A total of 11 groups submitted 30 runs. The following table and the three graphics below present the average classification score S obtained for each submitted run and each image type (scans, scan-like photos and photos). Click on the graphics to enlarge them. A last graphic presents the same results in a single graphic using histogram piles whose heights are computed by the mean of the 3 scores S. This gives an idea of the mean score over all image types while still keeping the relative performances on each image type. Additional statistics and comments on these results will be provided in the overview working note of the task published within CLEF 2012.
Run name |
runfilename |
retrieval type |
run-type |
Scan |
Scan-like |
Photographs |
Avg |
SABANCI OKAN run 2 |
1340914195368__SABANCI_OKAN_run1_PhotosWithManuelSegm |
Visual |
humanly assisted |
0,58 |
0,55 |
0,22 |
0,45 |
THEWHO run 3 |
1341076598230__THEWHO_run3 |
Visual |
humanly assisted |
0,43 |
0,4 |
0,49 |
0,44 |
SABANCI OKAN run 1 |
1340914029811__SABANCI_OKAN_run1 |
Visual |
Automatic |
0,58 |
0,55 |
0,16 |
0,43 |
IFSC USP run 1 |
1340646105047__IFSC USP_run1 |
Mixed |
humanly assisted |
0,35 |
0,41 |
0,51 |
0,42 |
LIRIS Reves run 1 |
1340967474781__LIRIS_ReVeS_run_01 |
Visual |
humanly assisted |
0,42 |
0,51 |
0,33 |
0,42 |
INRIA Imedia PlantNet run 1 |
1340800216179__INRIA-imedia-comb_matching_form-seg_filt_romb |
Visual |
Automatic |
0,49 |
0,54 |
0,22 |
0,42 |
INRIA Imedia PlantNet run 2 |
1340981884347__INRIA-imedia-shape_context-seg_romb |
Visual |
Automatic |
0,39 |
0,59 |
0,21 |
0,40 |
THEWHO run 4 |
1341086902847__THEWHO_run4 |
Mixed |
humanly assisted |
0,37 |
0,35 |
0,43 |
0,38 |
LSIS DYNI run 3 |
1340442006232__LSIS_DYNI_latefusion1 |
Visual |
Automatic |
0,41 |
0,42 |
0,32 |
0,38 |
THEWHO run 1 |
1341060086533__THEWHO_run1 |
Mixed |
humanly assisted |
0,37 |
0,34 |
0,43 |
0,38 |
INRIA Imedia PlantNet run 3 |
1341413130673__INRIA-imedia-multisvm |
Visual |
Automatic |
0,47 |
0,46 |
0,15 |
0,36 |
IFSC USP run 2 |
1340646169213__IFSC USP_run2 |
Mixed |
humanly assisted |
0,34 |
0,43 |
0,3 |
0,36 |
LSIS DYNI run 2 |
1340355731188__LSIS_DYNI_latefusion |
Visual |
Automatic |
0,35 |
0,35 |
0,29 |
0,33 |
ARTELAB run 1 |
1338909159599__ARTELAB-gallo-run |
Visual |
Automatic |
0,4 |
0,37 |
0,14 |
0,30 |
LSIS DYNI run 1 |
1340355500493__LSIS_DYNI_cdd_plants2012_lpq_spyr |
Visual |
Automatic |
0,33 |
0,32 |
0,24 |
0,30 |
THEWHO run 2 |
1341067995504__THEWHO_run2 |
Mixed |
humanly assisted |
0,3 |
0,25 |
0,24 |
0,27 |
LIRIS Reves run 0 |
1339772364611__LIRIS_run_00 |
Visual |
humanly assisted |
0,31 |
0,26 |
0,19 |
0,25 |
HFUT run 3 |
1340332545702__HFUT_Zhao_run3 |
Visual |
Automatic |
0,32 |
0,26 |
0,11 |
0,23 |
HFUT run 1 |
1340331426139__HFUT_Zhao_run1 |
Visual |
Automatic |
0,3 |
0,24 |
0,09 |
0,21 |
DBIS run 2 |
1340621257880__DBIS_format_run02_visual_qbe_998879 |
Visual |
Automatic |
0,27 |
0,17 |
0,17 |
0,21 |
DBIS run 1 |
1340621173682__DBIS_format_run01_visual_gps_qbe_204627 |
Mixed |
Automatic |
0,27 |
0,18 |
0,17 |
0,20 |
DBIS run 3 |
1340969891703__DBIS_format_run03_visual_gps_qbe_topk_150_250 |
Mixed |
Automatic |
0,24 |
0,16 |
0,16 |
0,19 |
BRAINSIGNALS run 1 |
1341325862488__BRAIN_SIGNALS_run1 |
Visual |
Automatic |
0,25 |
0,22 |
0,05 |
0,17 |
HFUT run 2 |
1340331843469__HFUT_Zhao_run2 |
Visual |
Automatic |
0,24 |
0,17 |
0,09 |
0,17 |
DBIS run 4 |
1340954813845__DBIS_format_run04_clustering_visual_gps |
Mixed |
Automatic |
0,23 |
0,14 |
0,13 |
0,17 |
IFSC USP run 3 |
1340978702513__IFSC USP_run3 |
Mixed |
Automatic |
0,2 |
0,14 |
0,12 |
0,16 |
LIRIS Reves run 2 |
1340963576839__LIRIS_ReVeS_run2 |
Visual |
Automatic |
0,21 |
0,15 |
0,05 |
0,14 |
RMIT run 3 |
1341068124404__RMIT_run3 |
Visual |
Automatic |
0,01 |
0 |
0 |
0,00 |
RMIT run 1 |
1341067956101__RMIT_run1 |
Visual |
Automatic |
0 |
0 |
0,01 |
0,00 |
RMIT run 2 |
1341068182642__RMIT_run2 |
Visual |
Automatic |
0 |
0 |
0 |
0,00 |
_____________________________________________________________________________________________________________________
Schedule
- 01.03.2012: registration opens for all CLEF tasks
- 26.03.2012: training data release
- 01.05.2012: test data release
- 15.05.2012: registration closes for all ImageCLEF tasks
15.06.2012 30.06.2012: deadline for submission of runs
- 15.07.2012: release of results
- 17.08.2012: submission of working notes papers
- 17-20.09.2012: CLEF 2012 Conference (Roma)
_____________________________________________________________________________________________________________________
Contacts
Hervé Goeau (INRIA-ZENITH, INRIA-IMEDIA): herve(replace-that-by-a-dot)goeau(replace-that-by-an-arrobe)inria.fr
Alexis Joly (INRIA-ZENITH): alexis(replace-that-by-a-dot)joly(replace-that-by-an-arrobe)inria.fr
Pierre Bonnet (AMAP): pierre(replace-that-by-a-dot)bonnet(replace-that-by-an-arrobe)cirad.fr